By Dr. Jonathan Shurlock
Edited by Dr. Ahmed El-Medany
Thanks to the relentless work of Professor Stephen Smith (@smithECGBlog), the concept of occlusive and non-occlusive myocardial infarction is becoming more widely used in place of the STEMI/NSTEMI paradigm. With this shift comes an increasing need for timely and accurate ECG interpretation, particularly when assessing for subtle changes.
Professor Al-Zaiti and colleagues have explored a method to support such ECG analysis through the development of a machine learning algorithm targeted at diagnosis and risk stratification for occlusive myocardial infarction (OMI).
The full open access paper should be read for a fuller understanding of the computational methods applied. In summary, the authors developed a convolutional neural network trained to identify ECG patterns corresponding to OMI, called the ECG-SMART score. The derivation (training) cohort included 4,026 consecutive patients presenting with chest pain, with ECGs that had already been labelled as OMI or Non-OMI. External validation was undertaken using two separate validation cohorts which included a total 3,287 patients.
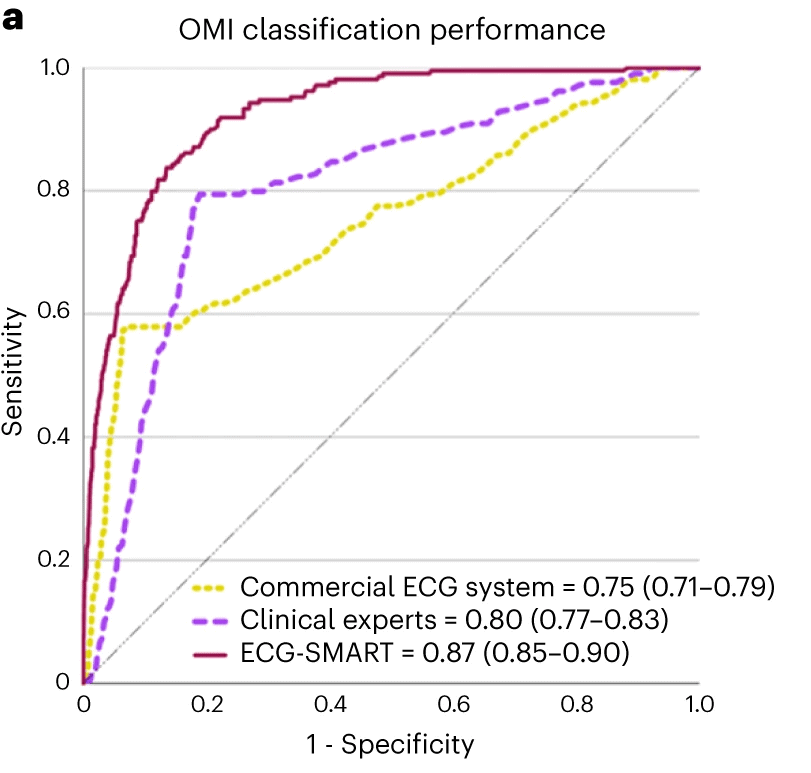
The authors demonstrated superior performance of their machine learning algorithm compared with clinical experts and commercial ECG interpretation systems, in the diagnosis of OMI (Figure 1).
As healthcare systems develop better understanding of the ECG changes representing occlusive coronary artery disease requiring urgent revascularisation, tools such as this are likely to support clinicians in making timely and accurate decisions. The ECG-SMART tool will benefit from wider testing over multiple centres and healthcare systems.
Read more here: https://www.nature.com/articles/s41591-023-02396-3